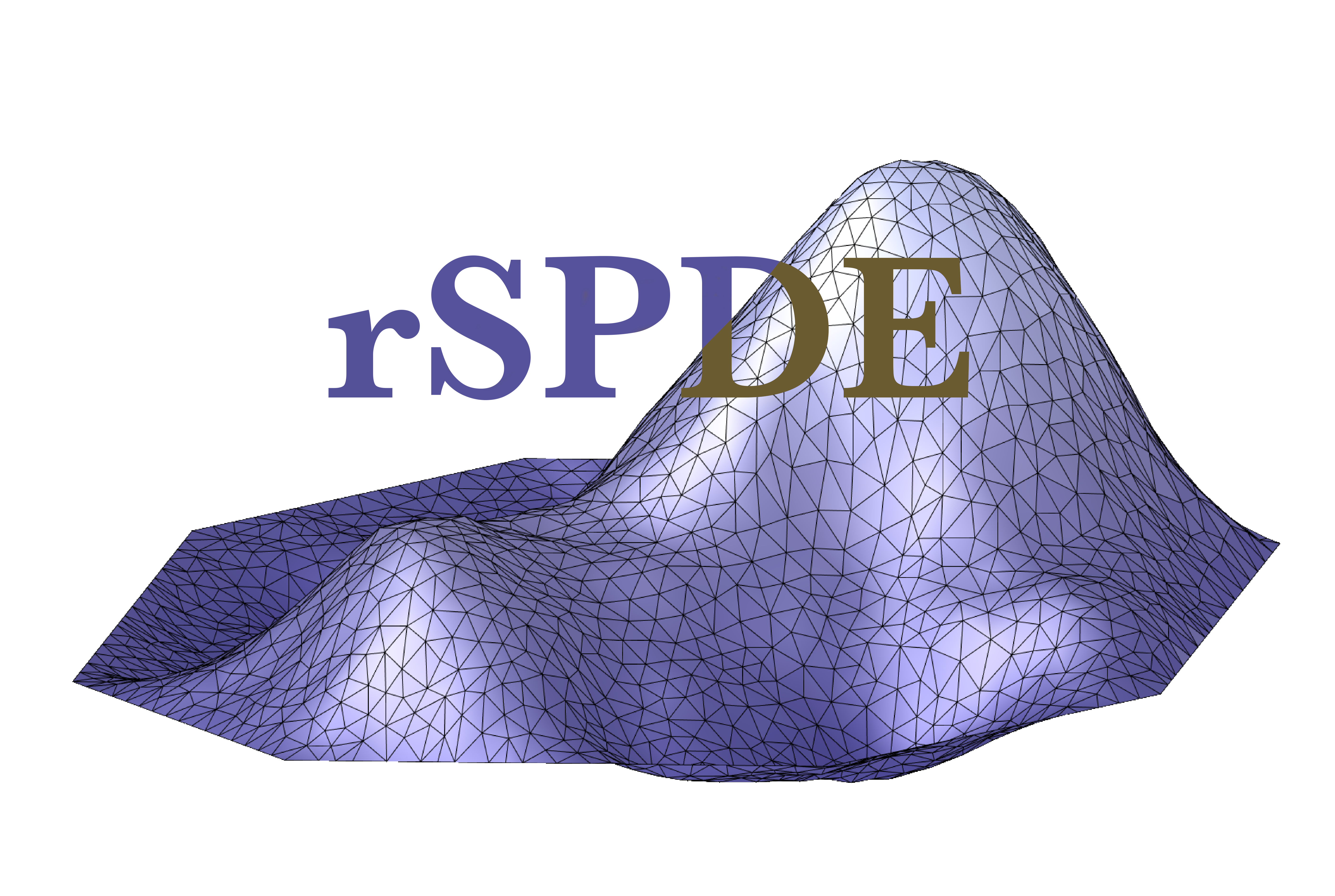
Constructor of Matern loglikelihood functions.
Source:R/fractional.computations.R
rSPDE.construct.matern.loglike.Rd
This function returns a log-likelihood function for a Gaussian process with a Matern covariance function, that is observed under Gaussian measurement noise: \(Y_i = u(s_i) + \epsilon_i\), where \(\epsilon_i\) are iid mean-zero Gaussian variables. The latent model is approximated using the a rational approximation of the fractional SPDE model corresponding to the Gaussian process.
Usage
rSPDE.construct.matern.loglike(
object,
Y,
A,
sigma.e = NULL,
mu = 0,
nu = NULL,
tau = NULL,
kappa = NULL,
sigma = NULL,
range = NULL,
parameterization = c("spde", "matern"),
m = NULL,
log_scale = TRUE,
return_negative_likelihood = TRUE
)
Arguments
- object
The rational SPDE approximation, computed using
matern.operators()
- Y
The observations, either a vector or a matrix where the columns correspond to independent replicates of observations.
- A
An observation matrix that links the measurement location to the finite element basis.
- sigma.e
IF non-null, the standard deviation of the measurement noise will be kept fixed in the returned likelihood.
- mu
Expectation vector of the latent field (default = 0).
- nu
If non-null, the shape parameter will be kept fixed in the returned likelihood.
- tau
If non-null, the tau parameter will be kept fixed in the returned likelihood. (Replaces sigma)
- kappa
If non-null, the range parameter will be kept fixed in the returned likelihood.
- sigma
If non-null, the standard deviation will be kept fixed in the returned likelihood.
- range
If non-null, the range parameter will be kept fixed in the returned likelihood. (Replaces kappa)
- parameterization
If
spde
, then one will use the parameterstau
andkappa
. Ifmatern
, then one will use the parameterssigma
andrange
.- m
If non-null, update the order of the rational approximation, which needs to be a positive integer.
- log_scale
Should the parameters be evaluated in log-scale?
- return_negative_likelihood
Return minus the likelihood to turn the maximization into a minimization?
Value
The log-likelihood function. The parameters of the returned function are given in the order sigma, kappa, nu, sigma.e, whenever they are available.
Examples
# this example illustrates how the function can be used for maximum
# likelihood estimation
set.seed(123)
# Sample a Gaussian Matern process on R using a rational approximation
nu <- 0.8
sigma <- 1
sigma.e <- 0.1
n.rep <- 10
n.obs <- 200
n.x <- 51
range <- 0.2
# create mass and stiffness matrices for a FEM discretization
x <- seq(from = 0, to = 1, length.out = n.x)
# Compute the covariance-based rational approximation
op_cov <- matern.operators(
loc_mesh = x, nu = nu,
range = range, sigma = sigma, d = 1, m = 2,
parameterization = "matern"
)
# Sample the model
u <- simulate(op_cov, n.rep)
# Create some data
obs.loc <- runif(n = n.obs, min = 0, max = 1)
A <- rSPDE.A1d(x, obs.loc)
noise <- rnorm(n.obs * n.rep)
dim(noise) <- c(n.obs, n.rep)
Y <- as.matrix(A %*% u + sigma.e * noise)
# \donttest{
# Define the negative likelihood function for optimization
# using CBrSPDE.matern.loglike
# Matern parameterization
loglike <- rSPDE.construct.matern.loglike(op_cov, Y, A, parameterization = "matern")
# The parameters can now be estimated by minimizing mlik with optim
# Choose some reasonable starting values depending on the size of the domain
theta0 <- c(
get.initial.values.rSPDE(mesh.range = 1, dim = 1),
log(0.1 * sd(as.vector(Y)))
)
# run estimation and display the results
theta <- optim(theta0, loglike,
method = "L-BFGS-B"
)
print(data.frame(
sigma = c(sigma, exp(theta$par[1])), range = c(range, exp(theta$par[2])),
nu = c(nu, exp(theta$par[3])), sigma.e = c(sigma.e, exp(theta$par[4])),
row.names = c("Truth", "Estimates")
))
#> sigma range nu sigma.e
#> Truth 1.000000 0.2000000 0.8000000 0.100000
#> Estimates 1.002128 0.1868185 0.9184139 0.100227
# }